On August 2nd, 2022, MindBridge’s Chief Technology Officer, Robin Grosset, and VP of Analytics and Data Science, Rachel Kirkham, hosted a virtual webinar on “Explainable AI, transparency, and trust.” The main focus of this webinar was to explore how business and audit professionals can rely on AI-produced data analytics results to make better decisions.
Thank you to everyone that attended the live event, and for anyone that missed it, you can view a recording of the webinar here or keep reading for a recap of some of the most valuable key takeaways.
Introduction
Artificial Intelligence (AI) is an exciting technology being adopted by numerous industries today. For example, in accounting and auditing practices, AI has the power to be transformational. Still, with increasing scrutiny on controls and audit approaches, some may be reluctant to adopt a technology they don’t understand. Explainable AI can help to mitigate some of these risks by helping users to understand analytics results and take action.
Data is the new oil, and AI is the new electricity.
The webinar started with Robin iterating the sentiment that data is the new oil and AI is the new electricity. Which he then further confirmed with a quote from the prominent AI/ML tech entrepreneur, Andrew Ng, who said, “Just as electricity transformed almost everything 100 years ago, today I actually have a hard time thinking of an industry that I don’t think AI will transform in the next several years.”
These two statements should help to set a precedent for understanding just how massive of a transformation AI will facilitate in everyday life. AI already powers many of our interactions today. Information technology, web search, and advertising are powered by artificial intelligence, and many more industries will soon follow suit. For these reasons, plus more we cover in this webinar, your AI must be explainable.
Legislative Landscape of Artificial Intelligence
Apart from the need for explainability, there is also a legal component where governing bodies are producing laws and standards around the creation and use of AI software.
EU GDPR Recital 71
A few years ago, GDPR came into force, and Recital 71 is an important yet often overlooked component. Recital 71 says that when it comes to any decision (that impacts a human) made based solely on automated processing, such processing is subject to suitable safeguards. These safeguards should include the right to specific information about the data subject and the right to obtain an explanation of the decision.
Montreal Declaration
The Montreal Declaration for a Responsible Development of Artificial Intelligence was announced on November 3rd, 2017. This set of ethical guidelines for developing artificial intelligence is the culmination of more than a year of work, research, and consultations with citizens, experts, public policymakers and industry stakeholders, civil society organizations, and professional orders. Included in the declaration is a Democratic Participation Principle, which states that “AIS must meet intelligibility, justifiability, and accessibility criteria, and must be subjected to democratic scrutiny, debate, and control.”
Often, this intelligibility tends to focus on the fact that AI must be intelligible to the people who create it; however, it should also be comprehensible to those who use it. This has been a part of the underlying principle that we have adopted at MindBridge from the very early days. We wanted to ensure that the people leveraging the outcomes of our AI can take advised action and understand what the AI is telling them.
The Black Box Problem
In computing, a ‘black box’ is a device, system, or program that allows you to see the input and output but gives no view of the processes. The AI black box refers to the fact that with most AI-based tools, we don’t know how they do what they do.
One of Robin’s observations while building AI for financial professionals and auditors, in particular, is that auditors are trained to be professionally skeptical. We have found this problem to be of immense importance when it comes to MindBridge and the adoption of our platform. “How do we solve this black box problem when the AI is a complex advanced analytics platform?”
We will go over some of these solution methods, but first, we want to point out some of the primary opponents to explainable AI.
“…we should be working hard to explain how AIs are constructed”
Detractors to Explainable AI
Geoff Hinton, known as one of the world authorities/fathers of AI, was quoted in Forbes magazine as saying that he thought that trying to explain an AI or requiring that an AI is explainable would be a complete disaster. However, we think he might have regretted making that statement because immediately, many experts jumped on board in disagreement, saying, “This is wrong, and we should be working hard to explain how AIs are constructed.”
Cassie Kozyrkov, Google’s Chief Decision Intelligence Engineer, has a blog where she talks about the circumstances around when we should expect an AI to be explainable vs. when we shouldn’t. One of her arguments for not making an AI explainable is that AIs are, or can be, as complex as a human brain. We don’t ask humans to explain their brain’s processes when making decisions, so it is unreasonable that we should have to explain AI. Fortunately for you, MindBridge sees this stance as a dodging rationale, made a bit too early, and that AI absolutely can be made explainable.
The detractors to explainable AI are essentially hung up on the deep learning neural network problem; however, there are many different approaches to understanding it.
MindBridge’s Explainable AI
From the very early days of MindBridge’s design, we have thought about how to construct an explainable AI and build it so that it can relate to the purpose for which our clients are using our system.
The idea behind MindBridge is essentially to generate insights about financial data. It shows you an aggregated view and allows you to drill into the detail of a single transaction with a low number of clicks. You can easily read the things in red in that single transaction to see why that transaction received a specific score. We break down all the causes and reasons, allowing you to go into more detail.
You can expand individual control points to get a detailed explanation of what it has found, why something was found, and even view scenes of how the algorithms work in plain language. You won’t find any sigma or chi-squared differential equations in our documentation.
We have invested a lot of time in breaking down all the different steps so that our clients can understand how the system works, what it can do, and what it finds. As a result, we have constructed an explainable AI, which is inherently explainable due to our ensemble AI approach and each control point in the ensemble being explainable.
Now, let us dive into some of the values behind this ensemble AI approach because it’s not just good at being explainable. It’s also good at being reliable and dependable.
MindBridge’s Ensemble AI Approach
There was a general principle that we started with at MindBridge, which was that we wanted to construct an AI system that would work in many different circumstances. Whether auditing a small firm, a multinational, a construction company, or a financial services company, we wanted to ensure that our AI always worked and provided relevant insights. And a part of doing that is not relying on a single model system.
Single model systems are quite common in the AI world; you train one model on a set of data, then use it to predict or select things for you. Instead, the idea behind our ensemble is that we use many different methods, i.e., design diversity, and then borrow from what is referred to as critical systems thinking.
There’s this notion of triplication if you need to depend on something and always need it to work. At MindBridge, we are going further than triplication; it’s not just three models. We started with seven, last year, we were at 28, and there are now 32 control points in our general ledger analysis. This approach provides multiple different control points, all combined to produce an overall signal or an overall view of potential risks within your data.
The MAYA Principle
Another philosophy we adopted at MindBridge is the MAYA principle which stands for “Most Advanced, Yet Acceptable.” In this approach, we combine traditional approaches, i.e., the conventional computer-aided audit tool approaches, with statistical methods and machine learning. We do this because we want to meet auditors and financial professionals where they are comfortable, which is this rule-based approach to finding interesting transactions. Then we layer statistical methods, like Benford’s law, or machine learning approaches, like our rare flow or outlier anomaly detection, on top of that traditional rules-based approach. This provides a side-by-side view so that you can see what machine learning can find and what the traditional rules-based approaches can find—all these act to offer unique insights into the data and a highly explainable system.
This approach becomes very actionable once you take all of these things and use them to understand the insights that the AI is putting out; very much an explainable AI with much capability that can help financial professionals understand what the AI is finding.
At this point, Robin hands the floor to Rachel to begin tying this all together around why you as an auditor should care and what you should do about it.
Anomaly Detection in Traditional Audit
When reviewing the journey of AI in audit, it’s clear that the adoption has been accelerating at a very fast pace. Five years ago, no one was using AI in live audits. Today, however, we can see that people are using artificial intelligence to help generate extra understanding of the data or to select their journals for management override or controls. In addition, these people are starting to generate substantive assurance by analyzing 100% of their transactions to assess the risk in different account areas. And when we expand into other kinds of datasets, moving from the general ledger to sub-ledger data, we start to generate even more evidence.
All this to say, AI isn’t going away. On the contrary, it’s gradually becoming embedded in how the audit profession works. Consequently, this also means that, of course, the regulators are taking notice.
When we think about “why is explainable AI important in audit,” there are a couple of different things to consider. For one, to ensure reasonable quality assurance, you must be able to generate evidence of what the AI told you about a particular population of data and be able to clearly articulate and document that.
There’s also increasing interest from the regulators in how audit firms verify what a system is doing. This interest has enabled new auditing standards, which refer explicitly to taking assurance over how software vendors operate. Further enforcing that explainable AI and audit can be the solution you need to improve your audits.
Explainable AI in Finance
Due to reluctance or lack of understanding of how AI works, the question always arises, “how am I going to get my finance team to adopt this technology?” However, when considering the impact of AI on different parts of the enterprise, it’s likely the same, if not greater, than the impact on audits. For example, AI can help you understand your internal control environment, detect where controls are operating effectively, enable better quality risk assessment over all your subsidiaries, move you towards a more continuous monitoring model of the finance team, and much more.
And if we consider being able to interpret results to have clear explanations of why a particular outcome has occurred, that helps cross that barrier to adoption as a finance professional.
That said, it is crucial to understand. So instead of taking a black box model to do a particular thing and blindly accepting the results, you should consider a few questions when vetting an AI solution.
- What am I implementing?
- What do I need to understand?
- What is the level of risk associated with the implementation of that algorithm?
- Are there any particular considerations that I have?
- How am I implementing the AI?
- What does it do?
- What data does it look at?
- What risks might arise from using that model?
Rates of Adoption in Finance
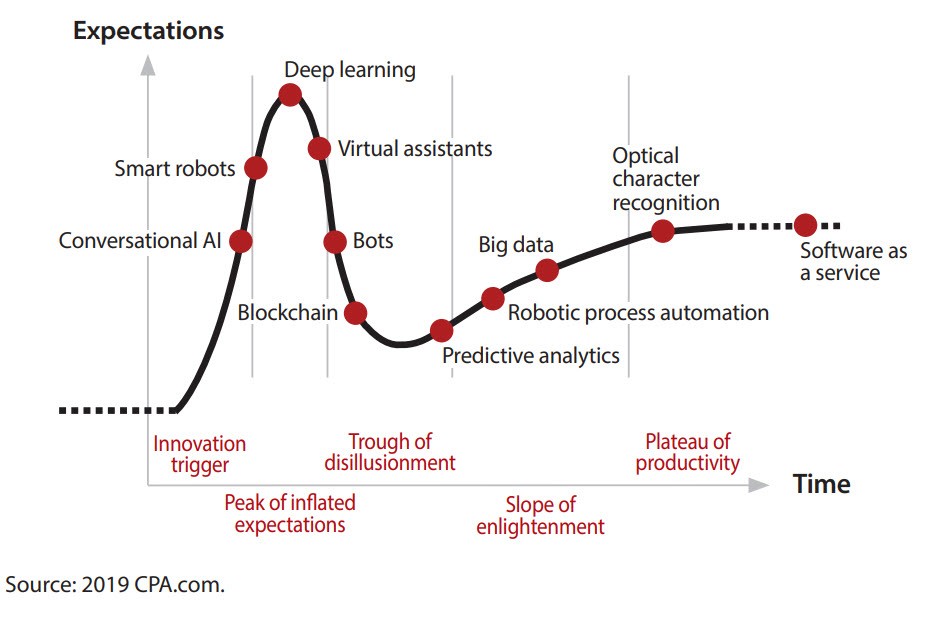
In this hype cycle, you can see how the adoption rate aligns with the broader adoption of technology across finance. Software-as-a-service has been adopted, many people have moved to cloud ERP systems, started using OCR for invoice processing, and large enterprises are generating ‘Big Data,’ particularly through the finance function.
When to Use Explainable AI?
Let’s face it; you should always use explainable AI. Of course, it is essential for the reasons mentioned above. Still, when viewing the landscape of interest in the space, you’ll notice a consistent theme in how people think about the widescale application of this technology.
The European Commission has published draft regulations over AI that will apply to everyone, whether you’re in audit or an enterprise. This will create a process for self-certification and government oversight of many categories of high-risk AI systems, transparency requirements for AI systems that interact with people, and even attempt to ban a few “unacceptable” qualities of AI systems.
Additionally, several different regulators in the UK have come out with a range of guidance on how to use AI. When is there potential for harm? How can you reduce the impact? And much like GDPR, the regulation of AI in Europe will have impacts worldwide.
International Standard on Quality Management (ISQM) 1
ISQM 1 strengthens firms’ quality management systems through a robust, proactive, and effective approach to quality management and applies to all firms that perform engagements under the IAASB’s international standards.
We wanted to highlight a particular section within the standard that pertains to how you make sure people are using the software effectively on the audit because it has a set of criteria that you need to consider when you’re developing, implementing, and maintaining an IT application.
For firms who plan on using a third-party provider to provide the API, there’s a whole set of questions you might want to ask your vendor and get some help documenting the approach they’re taking. For example, thinking about the software’s output, does it achieve its intended purpose? Do you understand the inputs? What quality controls do you have over the data inputs into that system?
And at MindBridge, we have prepared a complete set of responses to all of these questions. We understand the many regulatory pressures in the finance profession, and when we saw that ISQM 1 was coming, we entered into a third-party conformity assessment of our algorithms to help firms meet ISQM 1 requirements.
Building Trust in AI
As AI becomes more important in critical aspects of society, the need for trusted sources of AI grows. One way to do this is by checks and balances in the form of third-party assurance. In the case of MindBridge, we have done this by completing a comprehensive audit of our algorithms.
The independent assessment, conducted by UCLC (University College London Consultants), is an industry first, making it easier to rely further on the results of MindBridge’s artificial intelligence. In addition, it provides a high level of transparency to any user of MindBridge technology and assurance that our AI algorithms operate as expected.
This third-party validation and explainable AI and audit are two pieces of the puzzle in a growing trust in artificial intelligence results.
Live Demo
The webinar’s next portion comprised a demo of the risk overview dashboard within the MindBridge platform. It gave viewers a feel for the utilized explainable AI techniques and how they can help understand what’s happening in a financial entity.