To kick off 2023, Joe Welch, Global Implementation Lead, MindBridge, hosted a virtual webinar for financial and internal controls professionals titled “AI meets Internal Controls over Financial Reporting (AiCFR).”
The main focus of this webinar was to explore how AI can enhance your ICFR to be more efficient and responsive to organizational needs.
Thank you to everyone that attended the live event. You can view the webinar recording here or keep reading for a recap of some of the most valuable key takeaways for anyone that missed it.
Session host
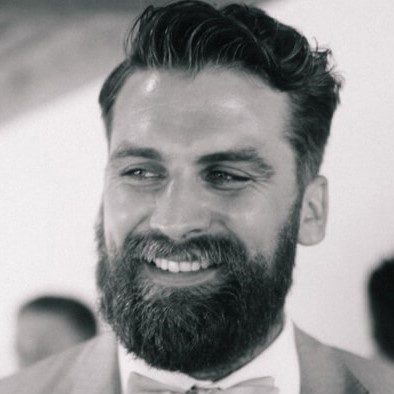
Global Implementation Lead
MindBridge
Joe is an ACA chartered accountant with over 10 years of internal audit and consultancy experience, specializing in the provision of risk management, internal controls, and data-driven assurance services across multiple industries.
What is ICFR?
ICFR stands for Internal Controls over Financial Reporting and refers to the controls and policies specifically designed to meet the following objectives:
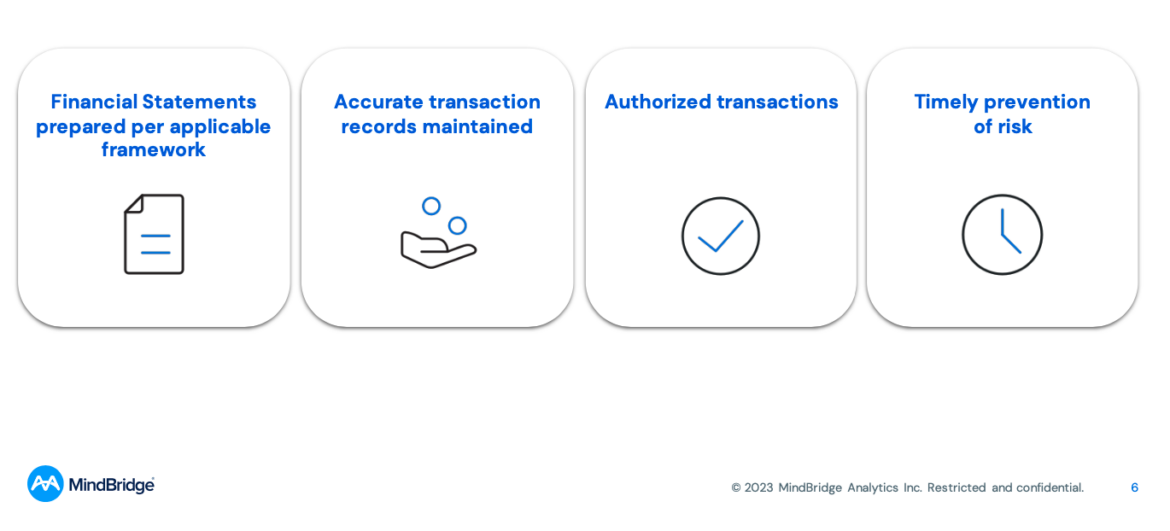
The first factor of ICFR is to ensure that financial statements are prepared per the applicable framework. Second, we must be sure the transactions are accurately recorded and retained. Next, ICFR is looking to ensure these transactions are authorized by the people charged with governance. Lastly, ICFR is in place to make sure that risk is mitigated in a timely manner and that any issues or known gaps identified are removed.
ICFR has always been an essential part of a CFOs agenda to ensure that the information they report in their financial statements, audit report, or annual report is accurate and doesn’t contain any material misstatement. However, it took time to reach this point.
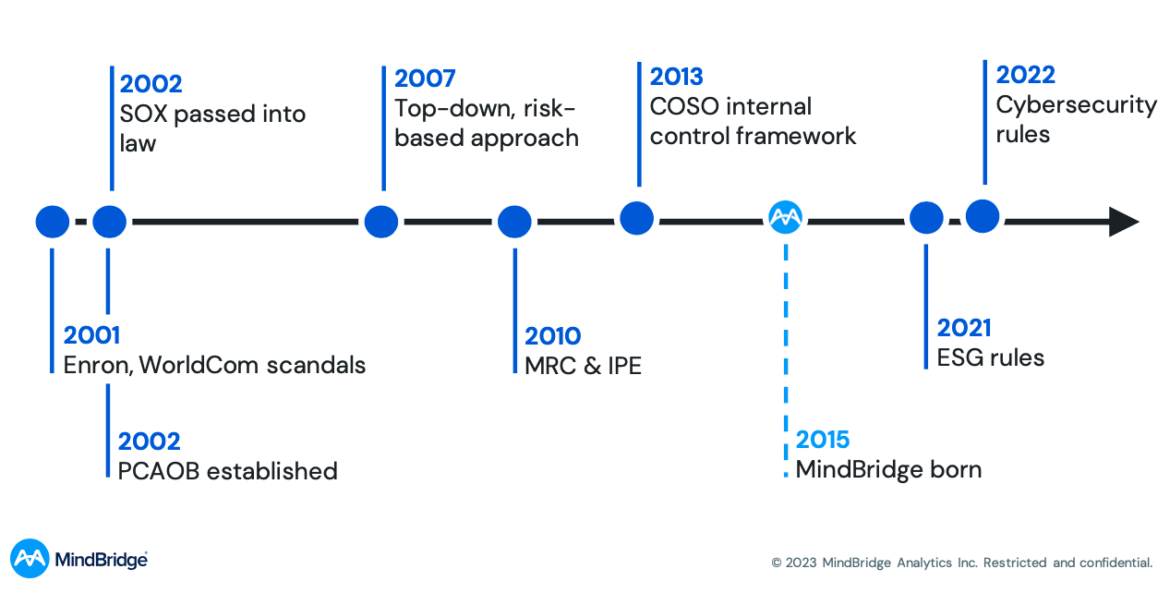
A few things in international regulatory reform are missing from this timeline. For instance, the UK has implemented UK Sox, a regulatory reform for all financial years ending in December this year and after. Other countries are also introducing reforms for internal controls over financial reporting. However, the tools available to help strengthen your ICFR and improve your internal controls have generally remained fairly constant.
Tools like Power BI may have improved the performance of internal controls, but they haven’t deepened the level of assurance that controls provide. And that’s where MindBridge comes into the picture.
MindBridge’s anomaly detection and financial risk discovery technology provide a deeper level of assurance. And with that, the focus switches to, “how can we incorporate such technology into your internal control framework?”
Dynamic Risk Landscape
Organizations and their transformation objectives are subject to a complex network of drivers of change, such as Sarbanes Oxley rules, regulatory rules, and COVID. This period of turbulence increases risk and the need for effective internal control frameworks to be effective.
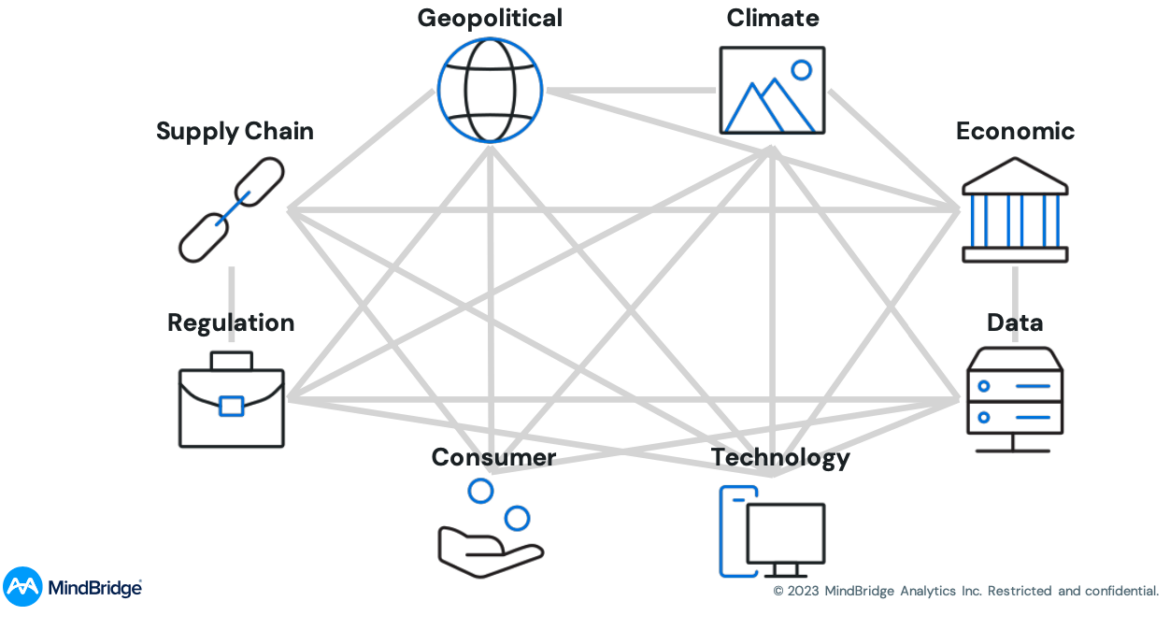
Anomaly detection technology
So, anomaly detection technology is the reason most of you are here and not just to learn about ICFR. Still, anomaly detection technology is the AI in AiCFR.
Organizations are deploying market-leading anomaly detection technology to respond to the dynamic risk landscape. This, combined with other internal control technologies, helps them enhance financial reporting objectives and maximize the value of their investment. The first we’d like to cover is human-centric artificial intelligence (HCAI).
Human-centric artificial intelligence
Human-centric Artificial Intelligence is a computer program designed to support and scale up human ideas and objectives rather than displace them. It relies upon and improves with human input, allowing AI to meet human objectives while preserving human control, especially in processes like ICFR.
For ICFR, HCAI’s collaboration between humans and AI is helping control owners reduce tedious workloads and get faster results. For example, AI-powered automation can now test full populations of data and prioritize the highest-risk transactions for closer investigation.
Machine learning
Machine learning (ML) is a type of AI whereby a computer program learns to recognize patterns with a dataset. This has enabled finance functions to gain a broader understanding of monetary flows and the rarity of general ledger account interactions on a periodic basis.
Leveraging this type of machine learning in ICFR is advantageous as it can save time by not requiring model retraining. In addition, unsupervised machine learning can also analyze data sets on its own and detect anomalies, which is more efficient than traditional methods.
Ensemble AI
Ensemble AI combines insights from multiple algorithms and tests to create a comprehensive risk assessment, similar to a panel of experts with different types of knowledge. It combines their findings to draw more robust and previously unknown insights about a particular transaction or data element.
An example of Ensemble AI being used in ICFR frameworks today is GL transaction risk scoring. Every journal entry in the ledger is simultaneously run through an ensemble of different tests (rule-based tests, statistical tests, and machine learning algorithms) to identify outliers and generate a risk score for each transaction.
Data validation
Data Validation is a type of anomaly detection technology that checks the completeness of data before running the anomaly detection technology. It includes detailed checks on every column of data to help find potential issues with the input file or data ingested.
Data accuracy is essential for financial professionals to make correct analyses. Control owners are now utilizing anomaly detection technology with built-in data validation to ensure data reliability and avoid wasting time on unreliable analyses.
AI meets ICFR (AiCFR)
So, you may be wondering, “how can I apply all of this to my internal controls over financial reporting?” The good news is that the ICFR framework can be mapped perfectly to one of the world’s most widely used frameworks for internal control: the COSO integrated internal control framework.
COSO defines internal control as a process managed by an entity’s board, management, and personnel to provide assurance that operational objectives, reporting, and compliance are achieved.
The COSO Framework is a system of 17 internal control principles across five components: control environment, risk assessment, control activities, information, communication, and monitoring activities.
Anomaly detection for a better control environment
The control environment is a set of standards, processes, and structures that organizations must implement and maintain to ensure internal control over financial reporting. It must demonstrate commitment to integrity, values, independence, oversight responsibility, structure, authority, accountability, and staff development.
Organizations have moved away from rarely using data analytics in their ICFR to redesigning their framework around anomaly detection technologies. This “data first” approach has created a structure for identifying, assessing, controlling, monitoring, and reporting financial and accounting risks. Further, organizations can attract and retain the right staff while setting a new tone from the top with a positive risk and control culture.
Anomaly detection for greater risk discovery
Organizations should perform risk assessments to determine the likelihood and impact of risks and the level of control needed to mitigate them. They should identify and analyze risks, assess fraud risk, and analyze significant changes period-over-period to ensure a sustainable financial reporting process.
Organizations are using ensemble AI to perform quantitative risk assessments of their data. Each financial transaction is assigned a risk score based on weighted rule-based tests, statistical models, and machine learning algorithms. The explainability of anomaly detection technology helps control owners understand what is driving risk in each transaction.
Transaction-based risk scores allow organizations to quickly identify the highest-risk divisions, operating units, functions, users, and financial statement accounts. It’s like a pivot table in Excel, allowing users to pinpoint and identify any risk in their organization quickly.
Anomaly detection for more effective controls
Control activities are procedures and policies that help management reduce financial reporting risks. They can be preventative or detective in nature and must take into account the principles of COSO to ensure effectiveness.
MindBridge is an anomaly detection technology that can replace manual detective controls in organizations. It can analyze 100% of transactions, giving a full view of data and insights into processes, allowing gaps in preventative controls such as restricting user access, workflow approval, segregation of duties, etc., to be spotted.
Anomaly detection for more relevant and timely information
Control owners must understand their roles in order to implement ICFR effectively. This component of COSO focuses on using and communicating relevant, high-quality information internally and externally to stakeholders.
One way to do this is with an API. An API, or Application Programming Interface, enables two software components to communicate with each other using a set of definitions and protocols. This can facilitate data uploads from ERP systems to anomaly detection platforms and export the insights and risk scores from the detection platform to GRC platforms, helping reduce risks, costs, and duplication of efforts.
Organizations using APIs experience significant time savings through automated control preparation and higher-quality information due to standardized results output.
Anomaly detection for simplified monitoring
Control monitoring is an evaluation process that ensures all control components of a framework are achieving what they are designed to do. Risk segmentation is one way MindBridge assists in this process by identifying and communicating any deficiencies.
Risk Segmentation is a useful tool for organizations to evaluate risk on a transactional level and begin their AICFR journey. It can be used for risk assessment or control evaluation and can be done on an ongoing or one-off basis. Organizations can then use technology to divide and view risk by different financial subprocesses, such as capital asset management and inventory.
Annotations and analytic annotations are also part of the ICFR process, allowing organizations to capture evidence of management review and ongoing monitoring. This evidence trail allows organizations to take credit for their anomaly detection as part of their control processes.
Starting your AiCFR journey
MindBridge advocates for customers to start their AICFR journey with General Ledger analysis. However, we are working with customers to build out processes on different financial data sets for a more holistic view.
MindBridge provides services such as receivables, payables, vendor analytics, payroll, and operating expenses. It can also help ingest and analyze transactional data to detect anomalies.
Companies should start by analyzing their general ledger data and use MindBridge’s risk assessment to identify gaps in their controls. They can also make their risk assessment a repeatable process and create controls built around a data-first approach in their framework. This will help them identify risks at the transactional level.
It’s important to understand that the technologies discussed in this webinar are available for users today. For more reading on this topic, Joe Welch has authored a whitepaper on AiCFR that goes deeper into each of the topics mentioned in the webinar.
If you have any questions about use cases, specific objectives, etc., book a demo or reach out to info@mindbridge.ai, and we will be more than happy to assist you.